Emergent Storage: a new avenue for neuromorphic computation
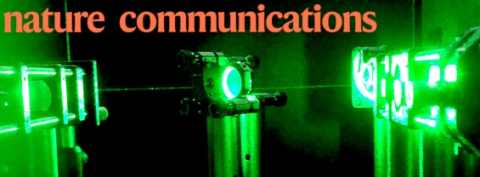
Artificial intelligence (AI) and deep learning are progressively reshaping various facets of our lives. However, a significant challenge associated with these advancements lies in the resource-intensive training phase, which demands substantial computational power and data, often consuming energy equivalent to that of a small town.Neuromorphic hardware, inspired by the human brain, presents a revolutionary computing architecture capable of substantially enhancing performance and energy efficiency in AI training compared to previous generations. Photonic architectures offer a promising avenue for further accelerating training processes while delivering lightning-fast operations.
In their recent paper titled "Photonic Stochastic Emergent Storage for Deep Classification by Scattering-Intrinsic Patterns," Marco Leonetti, Giorgio Gosti (from CNR nanotec), and Giancarlo Ruocco (from IIT-CLN2S and University Sapienza) introduced a novel neuromorphic architecture. This architecture demonstrates remarkable advancements by expediting both the training and operation of neural network classifiers while significantly reducing energy consumption.
The authors introduced a paradigm that draws inspiration from the human brain's concept of emergent memory storage, which facilitates the realization and storage of memory elements. This paradigm works analogously to our sensory system which encounters multiple imperfect replicas of objects from a class, and with an averaging process amalgamates these diverse patterns. Thus, from this averaging process emerges a nearly noiseless memory element that represents the class.
Similarly, the authors utilized random scattering patterns generated through a strongly scattering turbid medium illuminated by a laser. The emergent memory emerges by aggregating light from numerous noisy replicas leveraging the disorderly structures provided by nature to mitigate excessive noise.
This pioneering approach opens pathways to innovative photonic memory platforms, and it holds the potential to usher in a new era of machine learning with significantly reduced computational and energy costs in the training phase.
Paper:
Photonic Stochastic Emergent Storage for deep classification by scattering-intrinsic patterns
Marco Leonetti, Giorgio Gosti & Giancarlo Ruocco
Nature Communications 15, 505 (2024) DOI: https://doi.org/10.1038/s41467-023-44498-z